MOLP vs. DEA – Relatives or Friends
Pekka J Korhonen
Aalto University, Finland
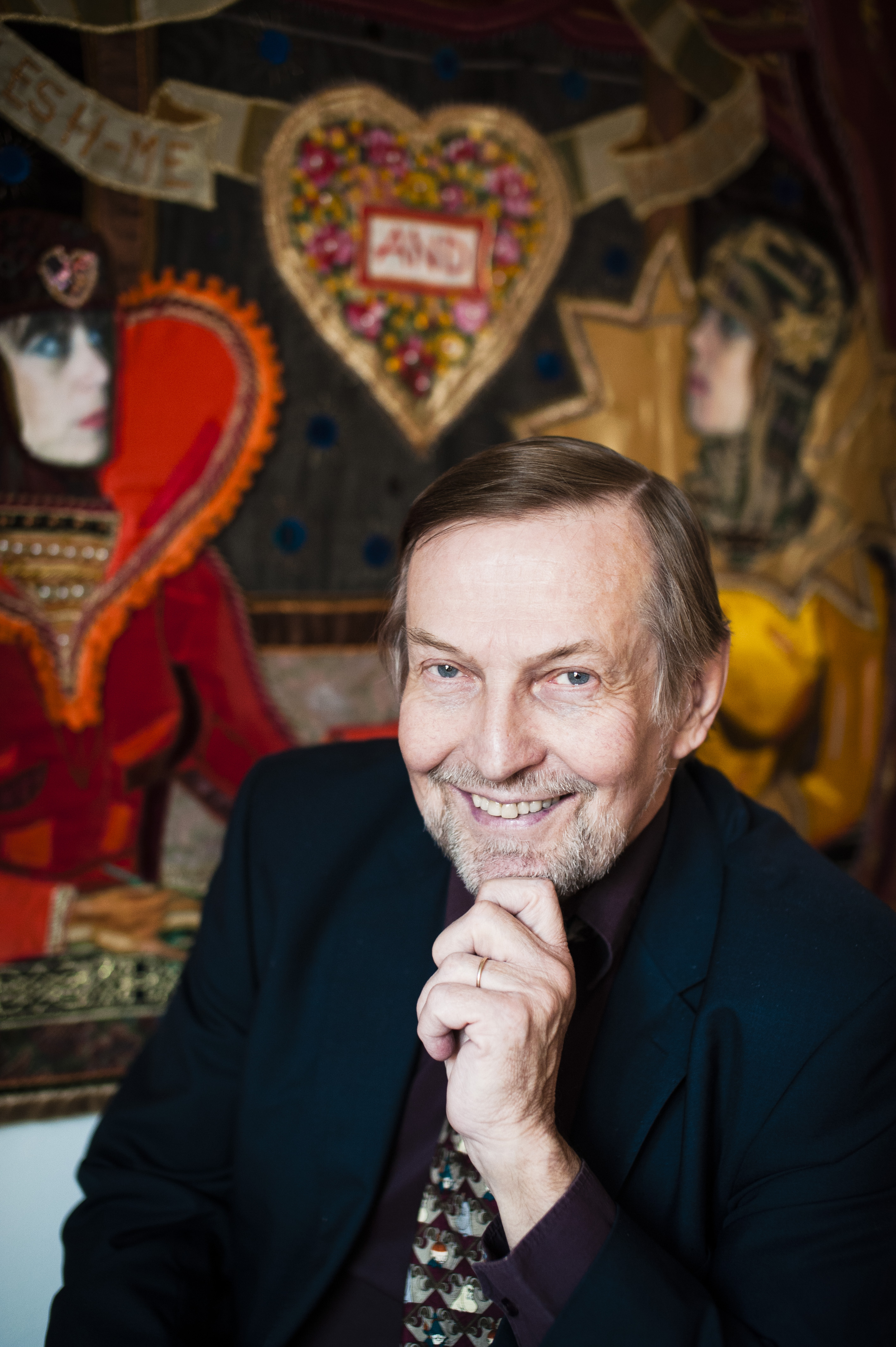
Abraham Charnes and William Cooper have had a significant impact on the development of Multiple Objective Linear Programming (MOLP) and Data Envelopment Analysis (DEA). The work of Cooper and Charnes on goal programming in the late 1950s can be regarded as pioneers of the MOLP-research area. Charnes et al.’s Management Science article from 1955 constituted the first goal programming model. The name “goal programming” was first introduced in Charnes and Cooper’s book: Management Models and Industrial Applications of Linear Programming, in 1961. It was many years a dominating way to solve Multiple Objective Linear Programming (MOLP) problems.
On the other hand, Charnes and Cooper are also the founders of the Data Envelopment Analysis. Together with Rhodes, they published the first article on Data Envelopment Analysis (DEA) in the European Journal of Operational Research 1978. DEA is characterized as a method for evaluating the relative efficiency of Decision Making Units (DMUs) performing essentially the same task. It has become very popular in practice and it is a hot research topic as well.
Although Charnes and Cooper have played a significant role in the development of DEA and MOLP, researchers in these two camps have generally not paid much attention to research performed in the other camp. Neither Charnes nor Cooper attempted to tie the two fields together. Even Cooper has said that MOLP and DEA are fully different analyses.
Despite many apparent similarities, DEA and MOLP (MCDM) research has developed separately. It is a pity, because —despite differences in terminology—DEA and MOLP address similar problems and are structurally very close to each other. In both models, technically speaking, the purpose is to identify efficient points in a certain space and suggest projections of inefficient points on the basis of such information. DEA and MOLP should not be seen as substitutes, but rather as complements.
In this tutorial, we show that the areas DEA and MOLP can cross-fertilize each other
Special Session sponsored by CDF
A tribute to Thomas L. Saaty & a great opportunity to learn ANP
Improve Your Decisions by Learning and Experiencing the ANP Best Practices
Orrin Cooper
University of Memphis, USA
Please bring your own laptop to this special session
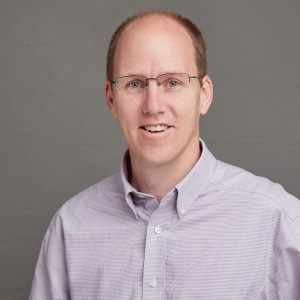
This tutorial is an Analytic Network Process (ANP) Best Practices experiential learning activity because we learn best by doing. Beginners with no prior experience will make their first ANP decision. Experts, and those in between the two extremes, will acquire valuable tools and techniques to improve the validity of their ANP decisions.
Attendees should come prepared with an interesting decision, big or small, that has at least 3 alternatives and 2 clusters, i.e. groupings, of criteria, with multiple criteria in each cluster to build a decision model during the tutorial.
The fundamentals of the ANP will be addressed, just in time, as we build our individual decision model. The ANP Best Practices will serve as our checklist to make sure that we address the critical components of an ANP decision. A review of ANP studies, that were published in the Social Science Citation Index (SSCI) over a one-year period, revealed that over half of the published ANP models had errors or omissions that were serious enough to bring the validity of the published model into question. These findings contributed to the development of the ANP Best Practices. The most common or critical omissions will be covered in greater detail at the specific times that they should be addressed when we are building our decision models. Tools and techniques will be provided to help decision makers: to check for the convergence of the Supermatrix, to identify and adapt a disjoint Supermatrix, to recognize and accurately capture the desired level of dependency in the Supermatrix, to test and improve the Coherency of the Supermatrix, and to conduct and report meaningful sensitivity analysis.
By the end of this tutorial, beginners will have “experienced” an ANP model from beginning to end; and experts will have more tools and techniques to improve the validity of their decisions.